Unlocking the Power of Annotation Tools for Machine Learning
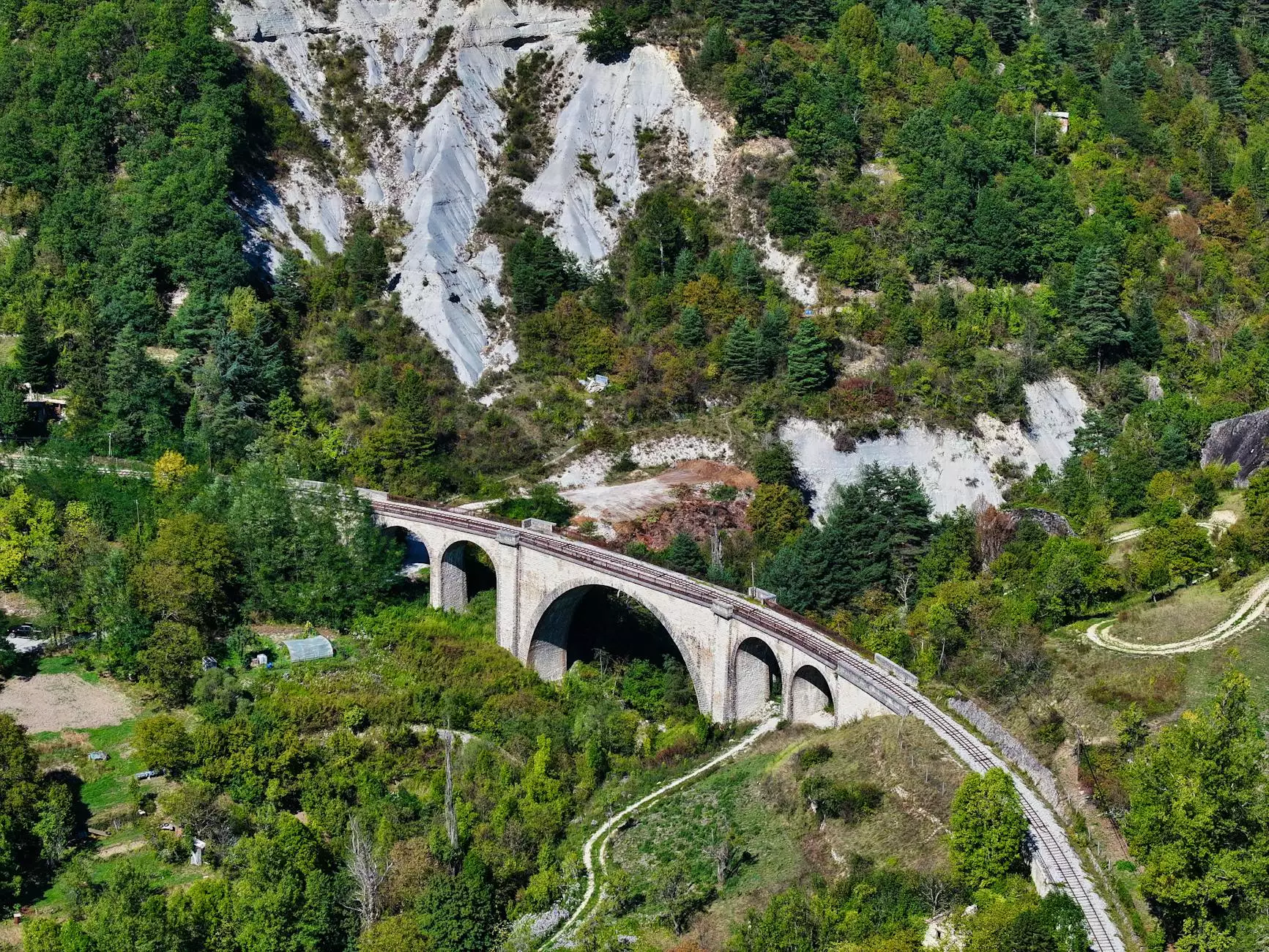
In today's digital landscape, businesses are constantly seeking ways to harness the power of data. One of the most transformative technologies in recent years is machine learning (ML), which utilizes algorithms and statistical models to analyze and draw insights from data. At the core of effective machine learning models lies an essential process: data annotation. This article delves into the significance of annotation tools for machine learning and how they can serve businesses, particularly in sectors like Home Services and Keys & Locksmiths.
Understanding Annotation in Machine Learning
Data annotation involves the meticulous labeling and categorizing of data in a way that a machine learning model can understand and learn from it. This process is typically performed on datasets that may include text, images, audio, and video. Machine learning models rely extensively on labeled data; thus, effective annotation is crucial.
Why Data Annotation Matters
Without accurate and high-quality data annotation, the performance of any ML model can degrade significantly. Poorly annotated data can lead to misleading insights, incorrect predictions, and ultimately, a failure to achieve the desired business outcomes. The importance of utilizing annotation tools for machine learning becomes ever more pronounced when considering the volume of data generated in various industries.
Types of Annotation Tools
There are several types of annotation tools for machine learning, each tailored for specific kinds of data. Here’s a look at some common tools and their applications:
- Image Annotation Tools: These tools add labels to images to help train computer vision models. They can provide bounding boxes, segmentation, or key points needed for tasks such as image recognition in home security systems.
- Text Annotation Tools: These are useful in organizing and labeling textual data for natural language processing tasks. For example, they can categorize customer service inquiries related to locksmith services.
- Video Annotation Tools: Essential for training models that analyze video footage, such as those used in surveillance.
- Audio Annotation Tools: These tools label audio data relevant to speech recognition and sound classification, beneficial for customer service AI.
Benefits of Annotation Tools for Businesses
The integration of annotation tools in business operations can lead to improvements in various areas:
1. Enhanced Data Quality
Using specialized annotation tools ensures that the data is labeled consistently and accurately, which increases the overall quality of the data fed into machine learning models. In home services, precise labeling can improve customer segmentation.
2. Cost Efficiency
While manual annotation can be time-consuming and costly, leveraging automated tools can drastically reduce the time required to prepare data for machine learning. For a locksmith business, this means faster deployments of AI-driven solutions to manage client inquiries or optimize services.
3. Faster Time to Market
With efficient annotation processes, businesses can quickly iterate and implement machine learning models. This rapid deployment can provide a competitive edge, especially when responding to market demands in home services.
4. Improved Customer Experience
By utilizing strong ML models powered by high-quality annotated data, businesses can personalize customer experiences, thereby enhancing service quality. For locksmiths, this could mean more accurate fault diagnostics or tailored recommendations.
Implementing Annotation Tools in Your Business
To successfully integrate annotation tools for machine learning into your business framework, consider the following steps:
1. Identify Your Needs
Before selecting annotation tools, assess what type of data you are working with and what your specific machine learning goals are. Understanding how these tools will fit into your business processes is essential.
2. Select the Right Tool
There are numerous tools available, ranging from open-source solutions to robust enterprise-level platforms. Evaluate their features, ease of use, and integration capabilities with your existing systems.
3. Train Your Team
Proper training on how to use annotation tools effectively is essential. Ensure that your team understands both the technical aspects and the importance of consistent, high-quality data labeling.
4. Monitor and Optimize
Keep track of the annotated data's performance and the machine learning models' results. Use feedback loops to continuously improve the data annotation process.
Real-World Applications in Home Services and Locksmiths
Understanding how annotation tools apply within your specific field can illuminate their potential. Here are ways these tools can be utilized:
1. Predictive Maintenance for Home Services
By annotating historical service data, machine learning models can predict when household appliances or systems will require maintenance or replacement. For instance, a locksmith service can predict higher repair calls based on seasonal trends from past data.
2. Customer Interaction Optimization
Using text annotation tools, customer service inquiries can be categorized and addressed more quickly. This allows locksmith services to respond to emergencies promptly while enhancing customer satisfaction.
3. Enhanced Security Solutions
Organizations can develop computer vision systems that monitor secure areas using video annotation. Labeling footage helps train ML models to recognize unauthorized access, thereby improving security measures.
The Future of Annotation Tools in Machine Learning
The scope of annotation tools is expanding with advances in AI and machine learning. Automated annotation methods, like machine-in-the-loop systems, are gaining traction, significantly reducing the labor involved in the process.
Technology continues to evolve, and staying ahead means embracing these innovations. As businesses in the home services and keys & locksmith sectors adapt, the integration of cutting-edge annotation tools for machine learning will prove pivotal for scalability and efficiency.
Conclusion
In summary, the world of machine learning and data annotation is rapidly developing, bringing forth unprecedented opportunities for businesses. By implementing high-quality annotation processes, companies can not only improve their operational efficiency but also enhance their customer engagement and service delivery. Investing in annotation tools for machine learning is not just a technological upgrade, but a strategic move towards a more data-driven future within the home services and locksmith markets.
Unlock the potential of your business by embracing these tools and elevating your approach to machine learning!